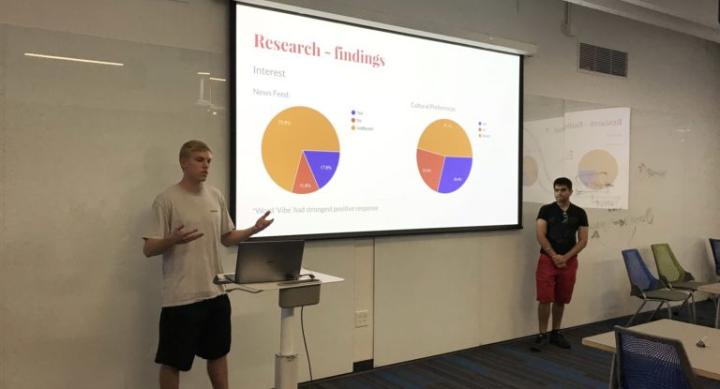
By Carly Burton, Center for Integrated Design Lecturer
In my last post I shared an overview of the design process and a few of the principles for artificial intelligence (AI) we generated in this fall’s Design and AI studio, a 5-week course aimed at exposing students to design as a tool for practical and meaningful integration of AI into our everyday lives. In this post, I’ll share how the student’s research insights influenced their design solutions, as well as share more on the AI principles created from their studies.
Human Insights that Influence
For their studio projects, students were asked to select a company and experience objective that would employ AI. In their inquiry they were to assess the company’s brand while also considering what would deliver value for the end consumer. After delving into foundational and generative research techniques, the students developed a design hypothesis that they took to target consumers. The objective was to enlighten and challenge their hypothesis for employing AI by better understanding the environment and individual that would engage with the solution. I’m a fanatical champion about this process because I argue it’s not about what technology can do and an idea; it’s about understanding people and the world in which they live. With that understanding, we can design meaningful products and experiences. Here are two examples of how insights influenced the studio project design solutions.
Hypothetical client: Whole Foods
Inquiry and Objective: What is the future wellness experience? Create a product or service using AI that can aid in improving individual wellness for Whole Foods’ customers.
Starting hypothesis: AI can improve customer wellness by providing intelligent, contextual advice during store visits, easing the shopping experience and enabling greater customer engagement and satisfaction.
Insight influencing solution: Shopping starts outside of the store.
Why this insight matters: Beginning the engagement once the customer is already in the store misses on the opportunity for intervention on consumption habits or influence on shopping routines. In addition, the solution should collect data outside of in-store purchasing behavior so that it can make more intelligent recommendations once at the point of sale. So to improve wellness for customers, the design solution must look at the total lifestyle of customers and intake data from sources outside of in-store purchasing patterns.
Hypothetical client: Airbnb
Inquiry and Objective: What is the future travel experience? Create a product or service using AI that enhances travellers experience.
Starting hypothesis: AI should deliver efficiencies. Users desire efficiency in using the application from browings to booking, and so Airbnb could streamline the user experience by using machine learning to improve a curated search process and utilize a chatbot that would allow users to search and confirm their bookings entirely through a messaging interface.
Insight influencing solution: There is a stark difference between being effective and being efficient.
Why this insight matters: Part of the delightful experience of Airbnb is the browse and discovery of your ideal destination and experience. Optimizing for efficiency by interfacing with a chatbot that masks a majority of the experience creates unease in users. Utilizing AI, however, to curate more relevant results that connect to the user’s needs creates a more effective browsing experience and in turn increased delight. So to enhance travellers experience, the solution should be focused on making effective recommendations personalized to the user, rather than a streamlined, narrow booking process.
In short, both of these are simple examples of how learning about human behaviors can influence the design and development of an experience that integrates AI.
Extracting principles for AI
The social inquiry also helped generate broad principles to guide the design and development of intelligent systems. As shared in the last post, the student’s studies pointed toward a recommended relationship hierarchy for AI and human actors. They also touched upon the importance of AI surfacing personally relevant content for beneficial human machine interactions. This desire for personalized experiences, which they felt was at the core of intelligent human machine interaction, came with some nuances:
First, they found that individuals were more willing for the machine to have access to more of an individual’s personal data if there was a clear direct benefit gained by the individual.
Second, there was a question of the relevance of data the machine was using to determine outcomes, so users should have some transparency into what data was being used to guide the interaction.
Third, they found that users would benefit from getting to “teach” the machine so that the interpretation of their data was accurate; so instituting a feedback loop would drive greater value and trust in the machine’s recommendations.
Last, as noted in the prior post, closing the system with only hyper-curated content/experiences could create adverse effects and limit possibilities; so they believed personalization should still introduce randomness.
A machine’s ability to achieve personalization for every individual may seem far-fetched; however, as more data is amassed on every part of our lives, how unlikely could it be that machines “know us” and curate our digital experiences to our liking? And what issues may arise for how we engage as individuals if our digitally mediated lives are all uniquely tailored to our desires?